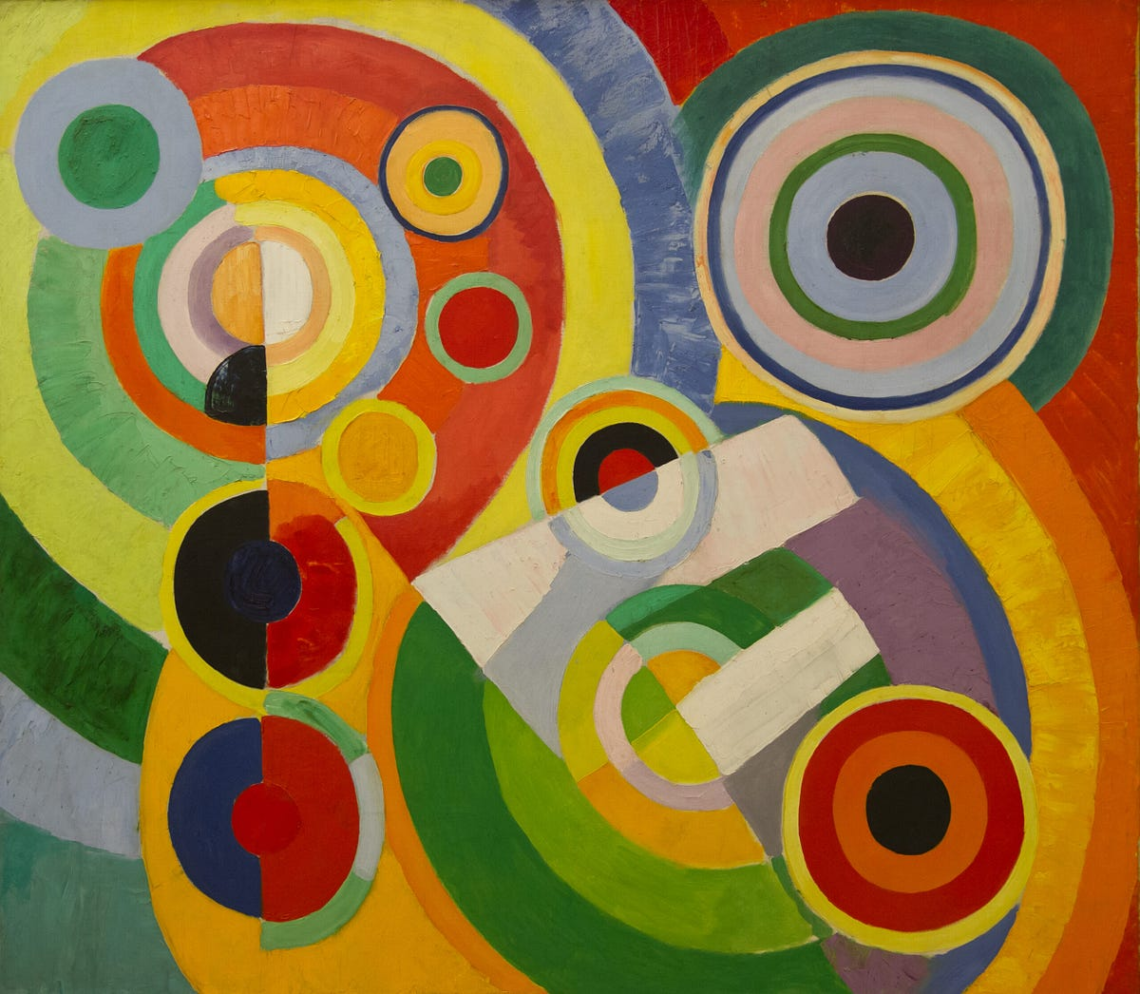
Predicting What Claude 3’s Position, on the LLM Leaderboard Reveals
Introduction
The advent of language models (LLMs) has transformed the field of natural language processing. These models have demonstrated power showcasing the ability to produce text resembling writing and comprehend intricate language structures. Among these models Claude 3 has gained attention in the AI community for its performance on the LLM leaderboard. In this piece we will delve into what Claude 3’s ranking on the LLM Leaderboard indicates about the future of language models.
The LLM Leaderboard
The LLM leaderboard is a platform that ranks language models based on their effectiveness across a variety of language tasks. Models are assessed using criteria like accuracy, fluency and coherence. This platform acts as a standard for comparing language models and monitoring their advancements over time.
Claude 3’s Ascendancy
Claude 3 has swiftly climbed to prominence on the LLM leaderboard consistently surpassing models in tasks. Its exceptional performance can be attributed to factors. Primarily Claude 3 benefits from a training dataset enabling it to draw insights, from sources and grasp the subtleties of language. Furthermore, Claude 3 utilizes cutting edge methods, like self-supervised learning and transformer architectures to improve its functionalities.
Implications for future
Claude 3’s ranking on the LLM leaderboard offers insights into the future of language models. Firstly, it showcases the significance of having access to training data. The data an LLM can utilize the better its ability to comprehend and produce text that resembles writing. This indicates that upcoming language models will see enhancements as more data becomes accessible.
Moreover, Claude 3’s achievements underscore the importance of employing techniques in developing language models. Self-supervised learning, which enables models to learn from data has proven to be a game changer in this domain. Additionally, transformer architectures, known for their capacity to capture relationships over distances have significantly contributed to boosting LLM performance. With these techniques continuously advancing we can anticipate remarkable language models in the time ahead.
Conclusion
Lastly Claude 3’s standing on the leaderboard prompts considerations about uses of LLMs. Given its skill in producing contextually relevant text Claude 3 holds promise for transforming sectors like content creation, customer service and language translation. As language models grow increasingly sophisticated and capable, we can expect their integration, into real world applications.
You May Also Like

5120x1440p 32:9 daisies images
July 7, 2023
rajkotupdates.news : upstox pre apply for an ipo via whatsapp
April 18, 2023